Labeling Tools for Machine Learning: Revolutionizing Data Annotation
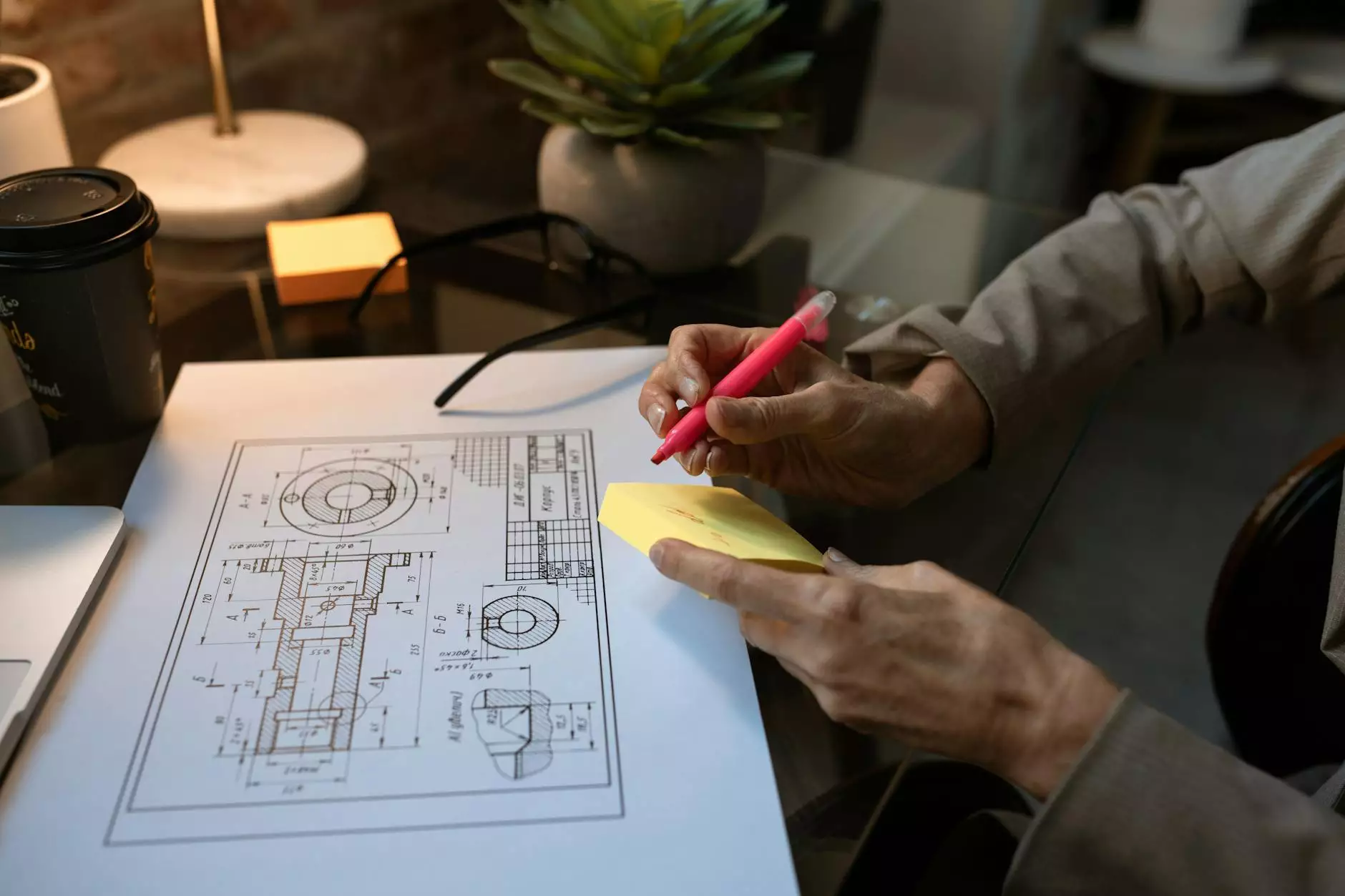
What Are Labeling Tools for Machine Learning?
Labeling tools for machine learning are essential software applications designed to aid in the annotation of data. These tools are pivotal in the training of machine learning models, providing the necessary groundwork to ensure robust and accurate AI systems. By converting raw data into meaningful labels, these tools empower organizations to optimize their machine learning workflows.
The Importance of Data Annotation in Machine Learning
Any machine learning project begins with data annotation, which is the process of tagging data with relevant labels to create a training dataset. Without this crucial step, models cannot learn from the data effectively. The importance of data annotation cannot be overstated; it directly affects the performance, accuracy, and effectiveness of machine learning models.
- Improves Model Accuracy: Accurate labeling leads to well-trained models capable of making precise predictions.
- Scalability: With effective labeling tools, organizations can handle large datasets more efficiently.
- Consistency: Automated tools help maintain consistent labeling practices across multiple datasets.
Types of Labeling Tools for Machine Learning
The landscape of labeling tools for machine learning is diverse, catering to various data types and use cases. Below, we explore the most common types of labeling tools utilized in the industry:
1. Image Labeling Tools
For projects focusing on image recognition and computer vision, image labeling tools provide functionalities such as bounding boxes, segmentation, and landmark annotations. These tools help in pinpointing specific objects within an image or categorizing entire images.
2. Text Annotation Tools
When dealing with natural language processing (NLP), text annotation tools specialize in tagging words or phrases to understand context and meaning. These tools allow for the identification of entities, sentiment analysis, and more.
3. Audio and Video Labeling Tools
For data that involves multimedia, audio and video labeling tools can annotate sound clips and video frames. They help in activities ranging from speech recognition to object tracking in videos.
Features to Look for in a Labeling Tool
Selecting the right labeling tools for machine learning involves considering several key features that enhance the annotation process.
- Ease of Use: The tool should have a user-friendly interface that allows annotators to work efficiently.
- Collaboration Capabilities: Look for tools that enable team collaboration, ensuring smooth workflows across projects.
- Integration Possibilities: The ability to integrate with other data processing and machine learning frameworks is critical.
- Support for Different Data Types: Ensure the tool supports various data formats based on your project needs.
Top Labeling Tools for Machine Learning
Here’s a curated list of some of the leading labeling tools for machine learning, each offering unique advantages:
1. Labelbox
Labelbox is a cloud-based platform that provides a robust annotation environment for images, text, and video. Its user-friendly interface makes it easy for teams to collaborate on labeling projects and manage workflows.
2. Supervisely
Aimed primarily at computer vision projects, Supervisely offers annotation tools alongside a powerful suite for managing datasets and training models. It is particularly well-suited for 3D point cloud annotations.
3. Prodigy
Prodigy is an annotation tool designed for NLP applications. It leverages active learning to streamline the annotation process by allowing machine learning models to guide human annotators in labeling tasks.
4. VGG Image Annotator (VIA)
VIA is an open-source tool that supports image and video annotations. It's lightweight and can be run locally, making it a good option for users who prioritize control over their data.
5. CVAT (Computer Vision Annotation Tool)
Developed by Intel, CVAT is an open-source annotation tool specifically designed for computer vision projects. Its features include support for a variety of annotation types and customizable workflows.
Best Practices for Effective Data Annotation
To maximize the efficiency of your labeling efforts, consider implementing these best practices:
- Define Clear Guidelines: Establish comprehensive labeling guidelines to ensure consistency.
- Use Pre-Annotation: Employ pre-annotations from AI models to reduce the workload for human annotators.
- Regularly Review Labels: Conduct audits and reviews of annotated data to maintain quality standards.
- Invest in Training: Provide your team with training on the tools and the labeling guidelines to enhance accuracy.
Challenges in Data Annotation
While data annotation is pivotal, it comes with its own set of challenges. Understanding these obstacles can help organizations prepare and adapt.
- Time-Consuming Process: Annotation can be highly time-intensive, requiring careful consideration of data context.
- Quality Control: Maintaining high-quality labels is crucial, and this can be challenging across large datasets.
- Cost Implications: Outsourcing annotation or employing a large team can increase costs significantly.
The Future of Labeling Tools for Machine Learning
As machine learning continues to evolve, so too does the landscape of labeling tools for machine learning. Future developments may include:
- Increased Automation: Advances in AI may lead to more automated labeling processes that require minimal human oversight.
- Real-time Collaboration: Improved collaboration features enabling real-time editing and feedback among team members.
- Integration with Machine Learning Pipelines: Seamless integration with model training pipelines to enhance the iterative process of machine learning development.
Conclusion
In conclusion, the significance of labeling tools for machine learning cannot be understated. They are at the heart of the data annotation process, enabling organizations to build reliable machine learning models. By selecting the right tools and implementing best practices, teams can enhance their annotation workflows, overcoming challenges while preparing for future advancements in the field. The right investment in labeling tools can set the stage for groundbreaking results in machine learning projects, driving innovation and delivering value across industries.