Image Annotation for Machine Learning: Transforming Data into Actionable Insights
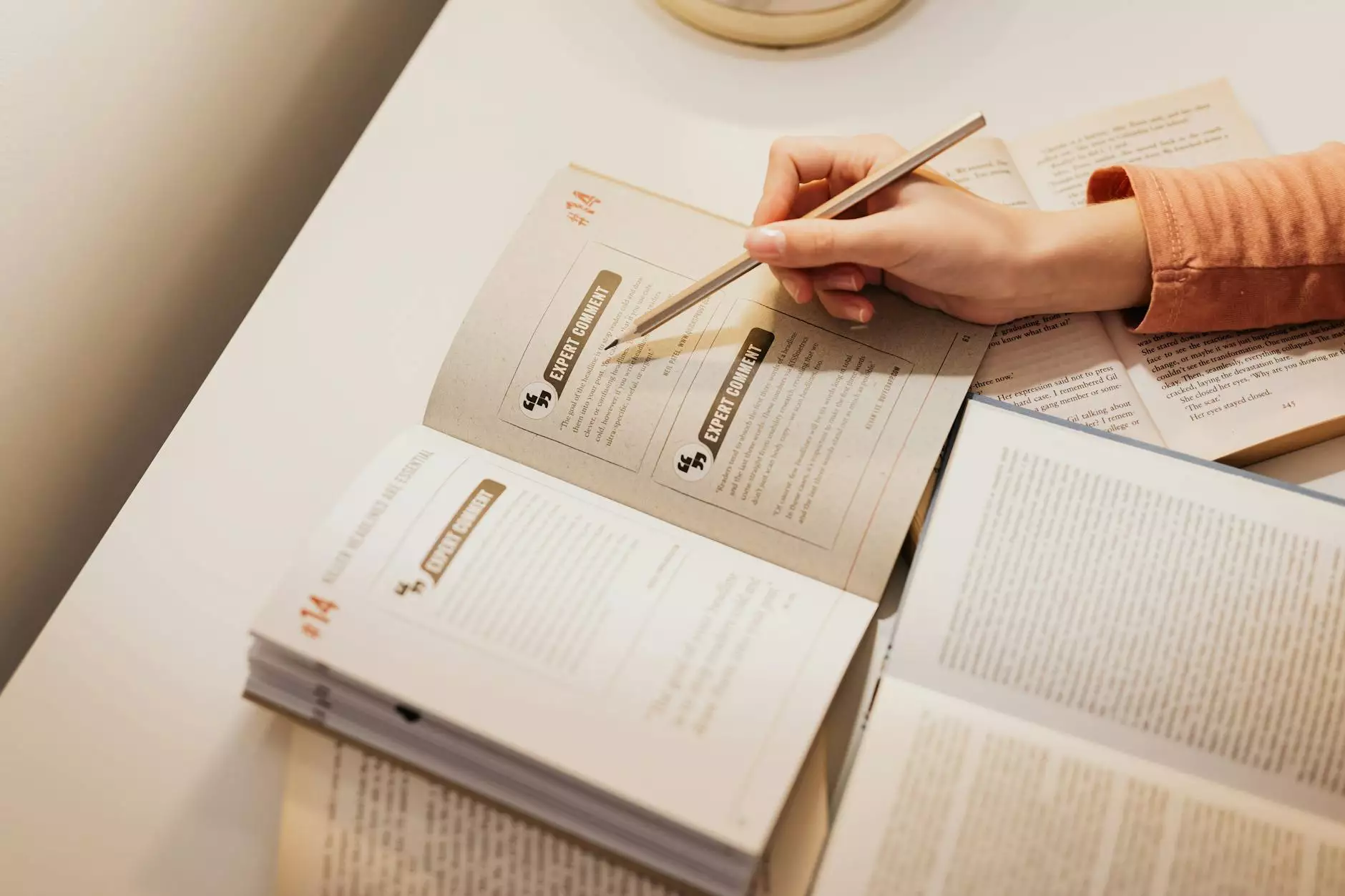
The explosion of data in the digital era has paved the way for advancements in machine learning and artificial intelligence. At the heart of these technologies lies image annotation for machine learning, an essential process that enables algorithms to understand and interpret visual content. As businesses strive to harness the power of their data, the demand for precise and efficient image annotation is at an all-time high. In this comprehensive article, we will delve deep into the significance of image annotation, its techniques, tools, and how platforms like keylabs.ai are revolutionizing this space.
Understanding Image Annotation
Image annotation is the process of labeling images to facilitate the training of machine learning models. By adding descriptive metadata, businesses can teach models to recognize various objects, patterns, and features within images. This labeled data is critical for supervised learning, where models learn from examples to make predictions or decisions without being explicitly programmed.
The Importance of Image Annotation in Machine Learning
Why is image annotation for machine learning so crucial? Here are several reasons:
- Enhanced Accuracy: Properly annotated images enable machine learning models to achieve higher accuracy rates by providing clear, contextual information.
- Real-World Applications: From self-driving cars to medical imaging diagnostics, accurate image annotation is foundational for numerous applications.
- Data Quality: Businesses that invest in quality annotation processes see a significant return on investment through improved model performance.
- Saves Time and Resources: Automated annotation solutions can drastically reduce the time and effort required to prepare datasets.
Types of Image Annotation Techniques
There are multiple techniques used in image annotation for machine learning, each suited to different types of tasks:
1. Bounding Box Annotation
This method involves drawing rectangles around objects of interest in an image. It is widely used in object detection where the goal is to identify and locate various objects.
2. Semantic Segmentation
In semantic segmentation, each pixel in an image is classified into a category. This fine-grained approach is essential for applications such as autonomous driving, where understanding every element of the environment is vital.
3. Instance Segmentation
Instance segmentation takes semantic segmentation a step further by differentiating between separate objects of the same class. This is crucial for accurately identifying multiple instances of, say, the same type of object in a scene.
4. Landmark Annotation
This technique involves placing markers on specific points of interest in an image. It's commonly used in facial recognition technologies to identify key facial features.
5. Polygon Annotation
Polygon annotation allows for more complex shapes than bounding boxes by drawing a polygon around an object. This technique provides a more precise representation of object boundaries.
Keylabs.ai: A Leader in Data Annotation Solutions
When it comes to image annotation for machine learning, keylabs.ai stands as a premier choice in the industry. The platform offers next-generation annotation tools tailored to meet the needs of diverse businesses. Here’s how keylabs.ai excels:
Advanced Annotation Tools
Keylabs.ai provides a suite of advanced annotation tools that support various annotation types, such as bounding boxes, polygons, and semantic segmentation. This allows users to customize their annotation process according to specific project requirements, enhancing both efficiency and accuracy.
User-Friendly Interface
The intuitive interface of keylabs.ai ensures that even those new to image annotation can navigate the platform seamlessly, making it accessible for teams of all skill levels.
Collaborative Annotations
Collaboration is essential in modern work environments. Keylabs.ai facilitates team collaboration, enabling multiple users to contribute to a singular project simultaneously. This feature boosts productivity and ensures everyone is aligned on project goals.
Quality Assurance Practices
Quality control is paramount in the image annotation process. Keylabs.ai employs rigorous quality assurance protocols, including automated checks and human verification, to ensure high-quality labeled datasets.
Scalability
As businesses grow, so do their data annotation needs. Keylabs.ai's platform is designed to be scalable, accommodating projects of all sizes without compromising performance.
Choosing the Right Image Annotation Platform
When selecting an image annotation platform, businesses should consider the following criteria:
- Versatility: The platform should support multiple annotation methods to cater to varied project requirements.
- Integration Capabilities: It should seamlessly integrate with existing data processing workflows and tools.
- Cost-Effectiveness: Consider the pricing structure to ensure it fits within your budget while providing the necessary features.
- Support and Training: Look for platforms that offer strong customer support and training resources to help teams get up to speed.
The Future of Image Annotation and Machine Learning
The landscape of image annotation is evolving rapidly, driven by advances in AI and machine learning technologies. Here are some emerging trends to watch:
1. Automation in Annotation
As machine learning technologies advance, the automation of image annotation is becoming more prevalent. Automated tools are being developed that can perform initial annotations with minimal human input, greatly speeding up the process.
2. Deep Learning Methods
Deep learning algorithms are increasingly being used to enhance the accuracy of image annotations, reducing the time needed for manual labeling.
3. Real-Time Annotation
With the rise of industries that require real-time data processing, real-time annotation technologies are being developed to provide immediate feedback and labeling as data flows in.
Conclusion
The role of image annotation for machine learning cannot be understated. It is the backbone of AI applications that require visual data interpretation. By leveraging powerful platforms like keylabs.ai, businesses can unlock the true potential of their data, gaining insights that drive innovation and growth.
By understanding the various annotation techniques, choosing the right tools, and staying abreast of emerging trends, organizations can position themselves effectively in the ever-evolving AI landscape. Investing in proper image annotation is not just a choice; it’s a commitment to excellence in the journey toward transformative AI solutions.